Temporal Semantic Correlation
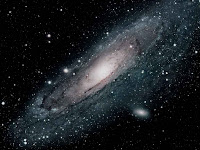
Semantic technologies are evolving continuously in their intended use and approaches.
For example, Semantic Analysis - the process of inferring meaning from the analysis of language - has been used traditionally to deliver a comprehensive synthesis of a potentially large number of complex documents.
More recently the focus of semantic technologies has been on inferring measurements of sentiments and predictions from Web 2.0 sources. In 1999 Tim Berners-Lee introduced the concept of a Semantic Web, a set of tools and technologies that produce a web of data that can be processed directly and indirectly by machines.
These include the Resource Description Framework (RDF), notations such as RDF Schema (RDFS) and the Web Ontology Language (OWL), all of which are intended to provide a formal description of concepts, terms, and relationships within a given knowledge domain.
While these semantic technology models allow for machines to understand information, they require the constant review of underlying assumptions and conventions. With the explosion of unstructured and unpredictable Web events emerging from anyone and anywhere, current semantic technologies are increasingly unable to deliver meaning and are limited to niche applications.
A new model is emerging that offers a complementary way to structure, observe, and gather meaning from time-sensitive chaotic information: the Temporal Semantic Correlation Model.
At the core of Temporal Semantic Correlation are the principles of chaos theory. Its application enables the self-organization of enormous amounts of data that coexist and constantly collide.
The social dimension of Web 2.0’s information explosion has created an environment where information exists in a chaotic, loosely structured repository. The more such information is created over a period of time, the higher the chance to observe patterns that reveal emerging themes. This leads to the observation of unexpected and timely concepts made visible through temporal correlation.
Darwin Ecosystem has developed and employs the Temporal Semantic Correlation Model in the development of its “Awareness Engine”. According to this model, the user does not need ask the system a direct, precise question. Instead, the self-organization of information is paired with the user’s context, delivering an interactive representation capable of informing, the user about what is happening about matters to them within their current context.
The Temporal Semantic Correlation Model delivers an organic semantic representation of concepts correlated among events over time. The model representation does not require and does not depend upon semantic analysis computing. It leverages Semantic Web tools to improve concept disambiguation consistent with the ontology familiar to, or inferred by, the user. Darwin Awareness Engine positions the user’s interpretation as the only judge of relevance.
The visualization of Temporal Semantic Correlation delivers and improves awareness and facilitates serendipitous discoveries. By observing patterns emerging from correlated terms, the effort of targeting and understanding relevant information is greatly improved at a personal level.
Darwin Awareness Engine is resilient to the evolving, diverse and growing sources of information since it does not require the information to be analyzed tagged, organized or curated. Further refinement however, is possible by using tools and specialized taxonomies that yield increased relevance within an emerging and progressively specific framework of conceptual nuances.
In summary, the Temporal Semantic Correlation Model complements semantic technologies that have been built upon the basics of how we think a machine should mimic our cognitive processes in order to infer meaning.
It turns out that it is necessary to include a less mechanistic approach; one where the technology seeks only to present patterns and relevance inherent within the mass of chaotic data. By affording more resilient and easier semantic refinement, Darwin Awareness Engine extends the user’s ability to infer meaning and relevance within a personal context.
Since our own thinking about what is important is highly influenced by our need to solve rapidly, evolving complex problems, today’s semantic technologies have difficulty maintaining continual coherence within our personal context at any given time. The maintenance of necessary deterministic rules is an ever-increasing and resource-consuming effort.
In contrast, the Temporal Semantic Correlation Model is consistent with nature’s self-organizing capability. This is a departure from the complexity of making the machine the “decider” of what matters or the arbiter of value in context. The discovery of valuable information and consensus-building recommendations, in this complementary approach, is left to the talented human. The system supports the human and not the other way around.
Comments